Finite Sample Properties of Statistical Estimators
Blog Post by Best Fin Investment
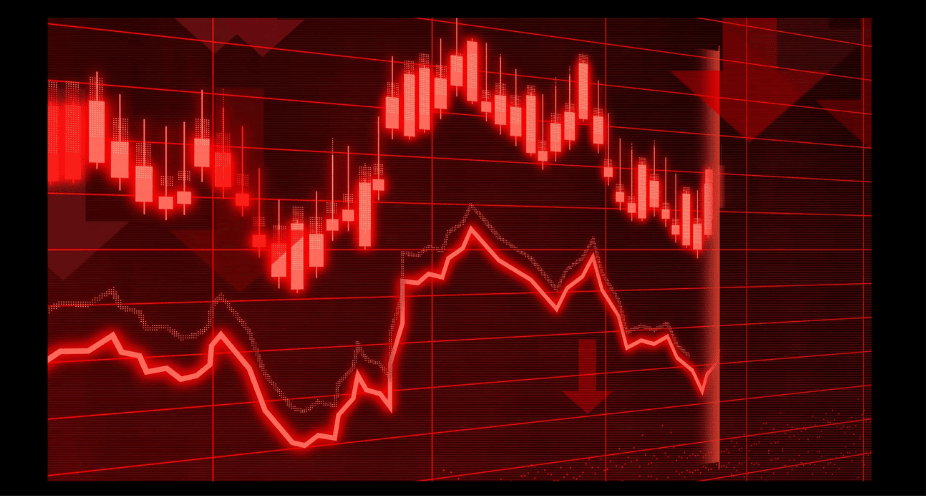
Table of Contents:
- Introduction
- Finite Sample Properties and Hypothesis Testing
- Example: Challenges of Applying Autocorrelation Functions (ACF)
- Conclusion
- References
- Related Articles
- Books from the References Section
- Additional Reads on Human Behavior and Behavioral Finance
Introduction:
In the realm of financial econometrics, hypothesis testing plays a crucial role in assessing the validity of models. Yet, the assumptions underlying many statistical tests often clash with the complex realities of empirical data. This incongruence prompts us to delve deeper into the finite sample properties of estimators, shedding light on their implications and helping to derive informed quantitative conclusions.
Finite Sample Properties and Hypothesis Testing:
In the hard sciences, such as in physics or engineering fields, we can conduct controlled experiments and in addition collect a very large number of observations (i.e. data points).
In some cases, such as in statistical mechanics, one may be able to conduct experiments and record up to 1020 data points! In such situations where sample size is very large, one can safely assume that the statistical estimator, defined as a sample average, is equal to the quantity it estimates, i.e. the unknown theoretical distribution (ensemble average).
Unfortunately economics & finance comes with two main limitations.
- First one cannot conduct controlled experiments, and testing ideas in economics isn't nearly as straightforward as it is in the hard sciences [3].
To circumvent this limitation, the standard approach in financial econometrics consists in conducting a so-called statistical test. We pretend that a particular hypothesis is true (what scientists call the Null hypothesis) and then ask what the chances are of seeing what actually happened given that hypothesis [3].
- Second, the number of observations in economics & finance is typically very small, i.e. we are dealing with so-called small-sample statistics.
Traditional hypothesis testing in financial econometrics delivers a statistic, (i.e. an estimate or forecast) together with its associated "confidence interval". This confidence interval is often derived by comparing test statistics to threshold values.
Now, it is well known that a statistic without a confidence interval becomes meaningless [1,4]. Indeed, as noted by Professor N. Taleb "It is not the estimate or the forecast that matters so much as the degree of confidence with the opinion" [5].
Unfortunately for the computation of confidence intervals, a large proportion of statistical tests necessitate specific assumptions to be met. For instance, residuals are typically required to be Independent and Identically Distributed (IID), and some of their higher-order moments, such as the fourth-order Kurtosis, must be well defined, i.e. finite [1]. Unfortunately financial empirical data are typically characterized by:
- Small-sample data sets: e.g. even five years of daily return is considered a small-sample when estimating the unknown theoretical distribution of the observations [1].
- Distributions having heavy and fat tails: typically referred to as "power law" decay.
- Nonlinear dependencies.
Hence the aforementioned characteristics of financial empirical data raise doubts about the meaningfulness of the various confidence intervals typically computed in classical financial econometrics.
Example: Challenges of Applying Autocorrelation Functions (ACF):
Autocorrelation Functions (ACF) have long been employed to adequately capture and analyze dependence in Gaussian time series and linear models [1]. Yet their suitability falters when applied to nonlinear, non-Gaussian time series prevalent in finance. For example, Davis and Mikosch's work [2] illustrates how heavy-tailed nonlinear time series can have non-standard statistical properties which invalidate many econometric testing procedures used for measuring dependence.
Hence, caution may be warranted when extrapolating insights from autocorrelations of price returns, or autocorrelations of nonlinear functions of price returns [1].
Conclusion:
The journey through finite sample properties of estimators and heavy-tailed nonlinear time series reveal the intricacies and potential difficulties associated with hypothesis testing in financial econometrics. As expressed by Professor B. Mandelbrot "If you are going to use probability to model a financial market, then you had better use the right kind of probability".
References:
[1] Cont R., "Empirical properties of asset returns: stylized facts and statistical issues", Quantitative Finance, vol. 1, issue 2, pp. 223-236, 2001.
[2] Davis R.A., Mikosch T., "Limit theory for the sample ACF of stationary process with heavy tails with applications to ARCH", Ann. Statistics, vol. 26, pp. 2049–2080, 1998.
[3] Lo A.W., "Adaptive Markets: Financial Evolution at the Speed of Thought", Princeton University Press, 2019.
[4] Mandelbrot B., Hudson R.L., "The (Mis)Behaviour of Markets: A Fractal View of Risk, Ruin and Reward", Profile Books Ltd , 2008.
[5] Taleb N.N., "Fooled by Randomness: The Hidden Role of Chance in Life and in the Markets", Penguin Books, 2004.